Reduce manual data preparations and technical debt with automation in risk analytics platforms
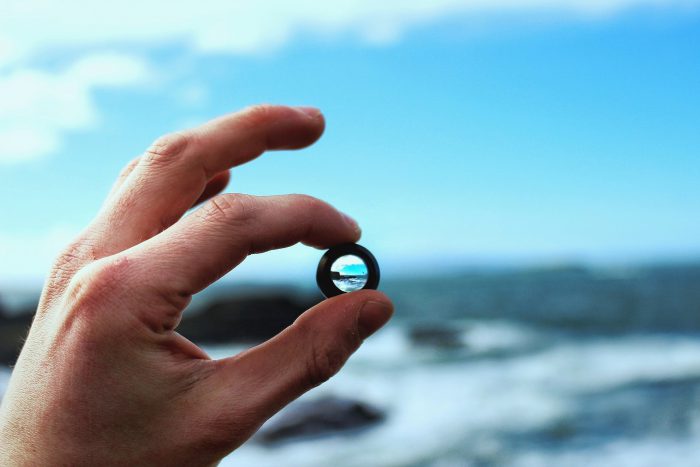
The nature of risk analytics will always equate to an environment of quickly changing demands. Unpredictability and volatility of external factors and a need for rapid and agile adaptation work against the need to produce accurate and informed forecasts. Delivering these results often forces enterprises to revert to manual interventions in input data generation processes.
But wait a minute: why are we still wasting our human effort on hand-tooling work arounds when we would rather apply our passion, talents, and unique institutional knowledge to make the most of our analytics assets? Sadly, the time we think we save with quick adjustments only introduce less-than-helpful human error and add up to technical debt with processes that are over-managed at the expense of real insight yields.
We all know how it begins. One simple adjustment to input data quickly becomes a series of manipulations and before you know it you have an entire work week or more devoted to manual preparation before you can run any models. Then comes the inertia stage when you’re forced to ignore or delay even more adjustments that you are certain will lead to more reliable forecasts. Whether it is tweaking a few cells or entire fields, you’re introducing layer upon layer of risk for human error.
One natural course-correction is adding rigidity to the input data preparation process. This also adversely impacts the human-driven insights that the process is intended to foster in your organization. The outcome: a reduced level of confidence in your predictions. Whether you or someone on your team is in the position to require manual manipulation of data, taking the step to eliminate past and future analytics technical debt and human error calls for a new habit: an automation-first approach to external data preparation.
Developing and implementing robust automation and change control systems can feel like an overwhelming and challenging endeavor, but the pay-off can be profound. Equipping a risk analytics team with tools that can efficiently handle a wide variety of change requests will instill greater confidence in the team and gain back more time for the organization to assess and react to ever-changing economic environments and demands. By removing the potential for human error, you create the open space required to leverage the human perspective.
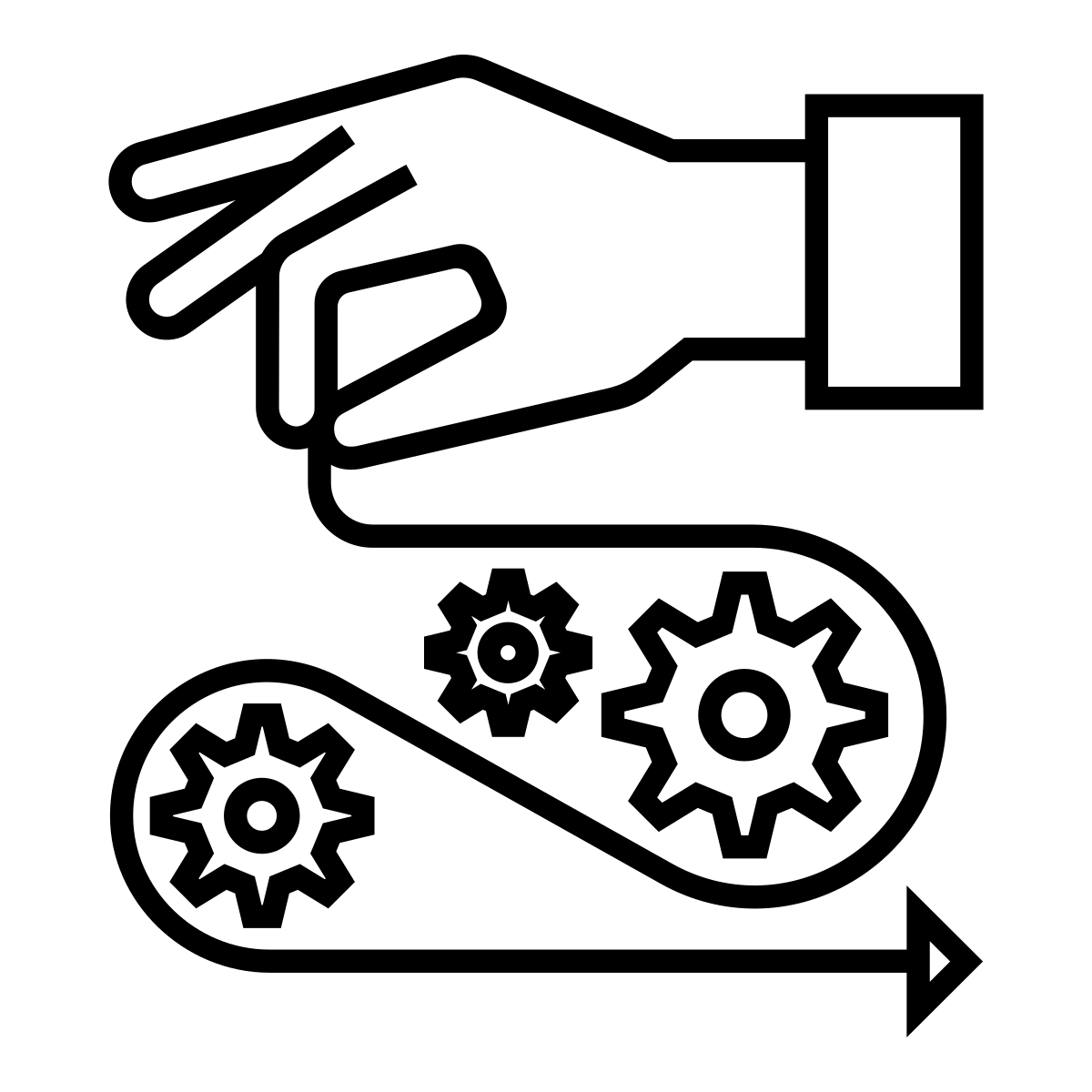
Automation replaces debt with know-how
Control and audit requirements for risk platforms make them particularly susceptible to technical debt accumulation. Working in rigid, inflexible platforms, many teams create and deal with technical debt, as well as accumulate technical debt over time. When a person or an entire team’s responsibilities revolve around adapting to manual workarounds, data preparation, and verifying they have enough evidence supporting these interventions to satisfy auditors, it’s time to assess how you value your team’s expertise and time.
Building platforms with automation in mind at each point of entry and exit can replace time spent working on the platform with time spent gathering evidence and insights. More time invested in the substance of data being ingested and how best to incorporate institutional knowledge about your data sources and portfolios into analytics can lead to higher model accuracy. Leveraging automation in risk preparations can both reduce the need for qualitative adjustments and their justifications, resulting in more polished and easily prepared deliverables supporting outputs such as earnings and disclosure reports.
As we discussed in our previous CRC blog, some preparation can be moved entirely out of the generation process by moving the adjustments into the models’ logic. Like it or not, there are going to be data prep processes for auxiliary data. This blog will focus specifically on economic data preparation as an example for automation, though we still see a wide variety of scenarios where manual data preparation will likely be the norm for the foreseeable future as part of continuing to run your models, including:
• Economic
• Coefficients – based on historical data
• Account attributes
• Validation
Common manipulations of auxiliary data
Most if not all models require supplemental economic data; you either leverage data from a source such as Moody’s or create your own forecasts. Risk analytics in the financial services industry rely on both historical and forecasted economic conditions whether from third party providers or your own enterprise. But the time it takes for these production models and data to reflect market changes will not always be sufficient for your organization’s reporting needs. Here are common reasons organizations manipulate auxiliary economic data:
• Blending scenario weightings
• Combining multiple sources into a single dataset
• Hand-picking scenario:mnemonic combinations
• Changing reversion periods
• Changing conversion methods of forecast periods
• Replacing expired variables/indexes
• Reflecting new crisis and/or upside scenarios (i.e. COVID, Russia/Ukraine conflict, other)
• Variable transformations
With such a broad swath of purposes – either with urgency or complexity attached – it’s not surprising that manipulation can be deeply entrenched in an otherwise rigid process. Staying swamped in the manual, dirty, and dull processes tied to external data preparation and the analytics required to generate insights is a recipe for failure.
Methods for automating economic data generation
Getting historical and forecasted macroeconomic data prepared for model runs can be tedious, and there are many situations that could require adjustment to the preparation process. These situations could include variable naming changes due to replaced or updated indices in data from a Moody’s or other similar subscriptions, new variables required for new or changed models, manipulations to business-as-usual forecasts at the variable and monthly level or changing vintages for specific scenarios. The list of use cases is a long, and with it can come the temptation to stay the course with ‘minor’ tweaks here and there make it fit your goal and timeline.
Picture this: instead of push back and excuses for not reacting to a situation, you’re reviewing activities in your applicable markets and coming up with appropriate response to unanticipated situations with meaningful adjustments that make your forecasts more reliable. What’s a good way to make that shift?
We recommend you start by automating the extraction process. Depending on your specific solution implementation, you may want to transform your variables for model usage before or after the economic data is loaded into your analytics platform. If your organization is getting economic data from a third party, we recommend integrating with that provider’s API. This will be the most efficient way to extract this data though some of our clients have been unable to get the sign-off from internal IT partners to configure this integration.
While it’s ideal and we work with clients to gain IT stakeholder participation, there are many ways to work around these technical challenges. In one circumstance we enabled the analytics team with utilities they could use on-demand to ingest final economic data inputs.
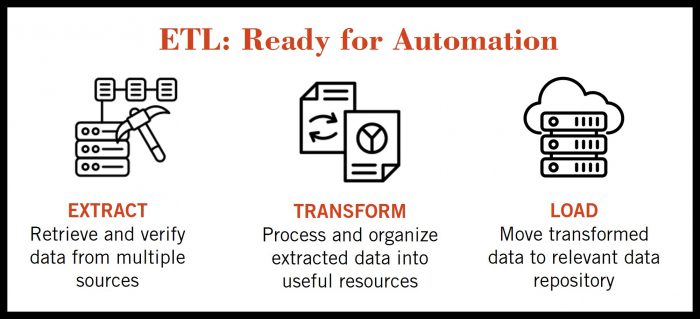
If you know the full scope of the changes you need and are willing to address, then building a user interface to apply these changes can be a great way to minimize time spent on change. However, rarely does an organization know the full extent of adjustments required to see meaningful impact. To accommodate the unknown, we find success in the balance between allowing some manual manipulations while still fitting requirements of automated processes. We may need to allow some work in Excel, for example, but the automated data generation process requires a specific data model, incorporates error handling, produces the data required for downstream models with reliability, and records necessary audit and control information. With these modifications in place the time spent preparing and validating the adjustments is reduced to minimal human effort.
Reep the rewards of automation
Automating data generation and modification processes is a great way to stay nimble, engaged, and innovative. Running a successful financial services organization requires informed decision making. We can never anticipate every question that will need to be answered, so long term success of informed decision-making processes is founded on both efficiency and agility.
Think of all the context you could add to presentations and reports if your time was valued more for gathering evidence as opposed to just preparations. It could be as simple as a phone call into someone in finance or accounting to better understand what bankers are seeing in the field with their customers or calling a peer at a different organization to understand how they’re planning to address a similar situation.
The alternative reads like this: In the case of one client who hired a recent masters graduate, the majority of job responsibilities quickly turned into keeping track of all the versions of a single economic dataset that the organization was evaluating on a monthly basis. The manual nature of the processes they set up around tracking, analyzing, and manipulating this single component of input data, led to preventable errors, and extra time spent triple-checking their work. Needless to say, it wasn’t the dream of the new hire or the outcome the company hoped for when they expanded the team.
When you free up time for people to engage with the specifics relating to risk forecasting that spark their areas of interest and true enjoyment, the results will delight you and your organization.
Adjustments are the constant
There will always be unforeseen circumstances that impact your modeling needs. Where the standard data requires manipulation, even a simple template set up for incorporating controlled adjustments to economic input data will set your team up for success.
Global events such as the COVID-19 pandemic and the Russia-Ukraine conflict, national interest rate changes, or even weather or industry-related metro-level events shouldn’t require platform limitations into how you want to handle these unforeseen market/portfolio changes. There are methods for adapting to these circumstances in the most appropriate manner and with confidence that the desired approach was implemented completely and accurately.
For example, we may need to incorporate economic scenarios that are specific to one or more of these events, that are out of the typical scope or subscription of activity. By setting up automated economic data preparation for our clients, our partner organizations handpick specific variables and/or blended multiple versions of these scenarios into their exposure forecasts allowing for the most appropriate action to take at that time. This saved them time and risk in their qualitative factor adjustment processes.
Don’t forget the breadcrumbs trail: auditing and logging musts
An additional and critical component of a successful automated process is the capability for leaving ’breadcrumbs’ with information such as:
• Run dates and times
• Completion dates and times
• User(s)
• Full logging of data sources, business logic, and output data
• Automated reporting/analysis of input and output data
When you can answer important questions about the analytics and/or data origins – who ran the process, when did the process run, when was the last run, with what parameter settings – with system-generated outputs that offer greater peace of mind knowing you covered your bases from an audit perspective.
Are you ready to automate?
Making the shift from manual data prep to a more automated approach isn’t a one-and-done exercise; great organizations are continually watching for signals in the market that they need to factor into their business and risk strategies. But as the saying goes the change doesn’t happen without finding a place to start. A good risk analytics team is motivated first and foremost to automate where the burden of manual oversight is replaced with the joy of adding their human point of view on data for insight and innovation. And a great risk analytics organization will only get better over time as they gain confidence in their ability to automate where the gains are greatest and manipulate only where absolutely necessary. It is important to maintain balance between flexibility, control, and automation, because when your organization is nimble, reducing the time required for implementing improvements will help keep the human perspective alive in analytics.
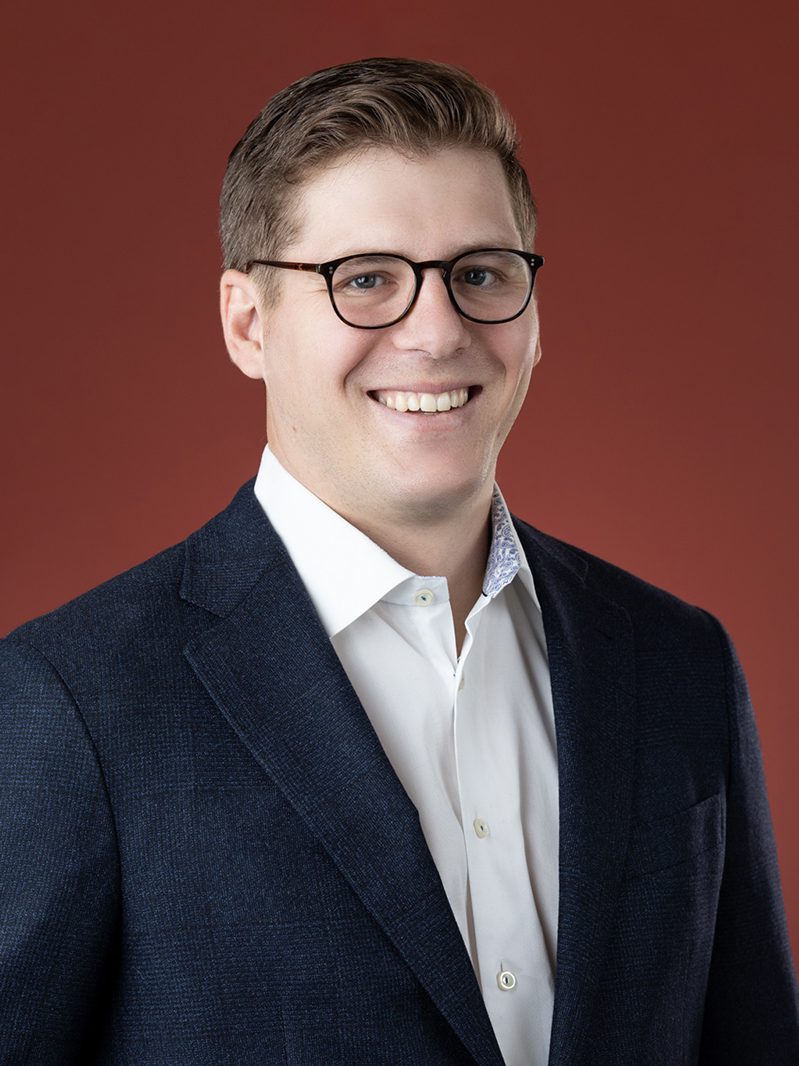
The intersection of math, economics, and athletics spark Austin’s passion for analytics in the world of finance. Austin is passionate about advising and implementing modernization and risk analytics strategies that perform well and enable organizations with the tools and understanding necessary to meet the dynamic demands of modern data and analytics. Connect with Austin on LinkedIn or connect with Corios to kick off a project.